AI detects lung diseases from the tiniest of ultrasound details. Generated by DALL-E –
The model, developed by researchers at Australia’s Charles Darwin University (CDU), United International University (UIU) and the Australian Catholic University (ACU), can identify specific patterns of different lung disease, outperforming previous AI tools that have been tested on the same ultrasound datasets.
“The model also uses AI techniques to show radiologists why it made certain decisions, making it easier for them to trust and understand the results,” said study co-author Niusha Shafiabady, a professor at CDU. “This model helps doctors diagnose lung diseases quickly and accurately, supports their decision-making, saves time, and serves as a valuable training tool.”
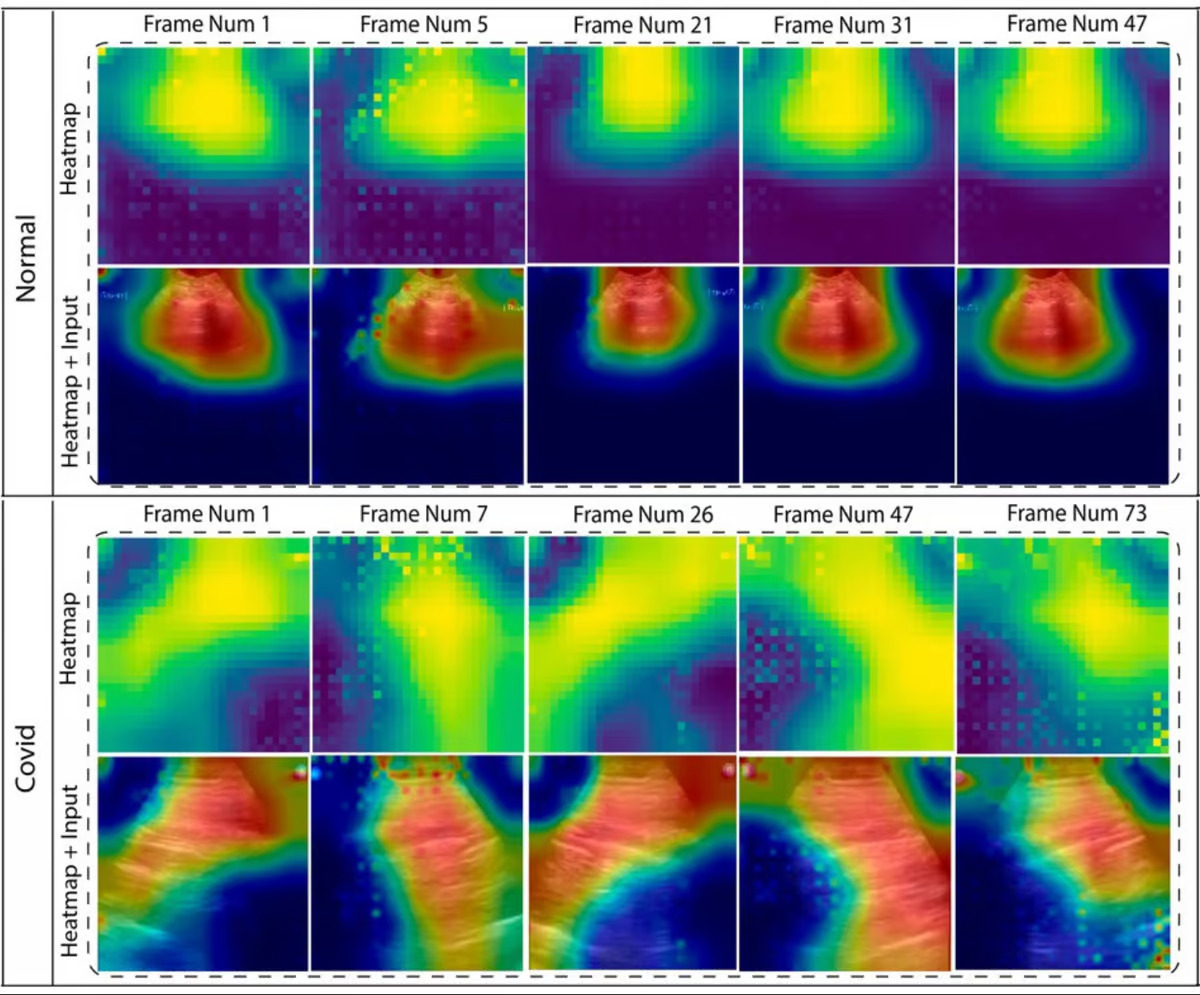
The team combined two kinds of AI models, highlighting how adaptable the technology is for diagnostic needs. One, known as a convolutional neural network (CNN), seeks out patterns in images or frames, focusing on the tiniest pixel-based changes that the human eye can miss when examining scans. Then, a long short-term memory (LSTM) model uses this information and puts it into a broader context, analyzing the CNN’s data over time while ‘forgetting’ irrelevant data.
With their powers combined, the novel hybrid model known as TD-CNNLSTM-LungNet is able to pick up abnormalities incredibly well – and then explain what the issue is. What’s more, it can determine if the scans show evidence of pneumonia, COVID-19, other lung diseases or if the lungs are normal. With a high ‘recall’ rate of 96.51%, this essentially means there are very few false negatives identified by the AI – which is important in treating time-critical lung conditions.
Using ultrasound videos of existing datasets, the model surpassed existing AI diagnostic tools, which currently score around 90-92%.
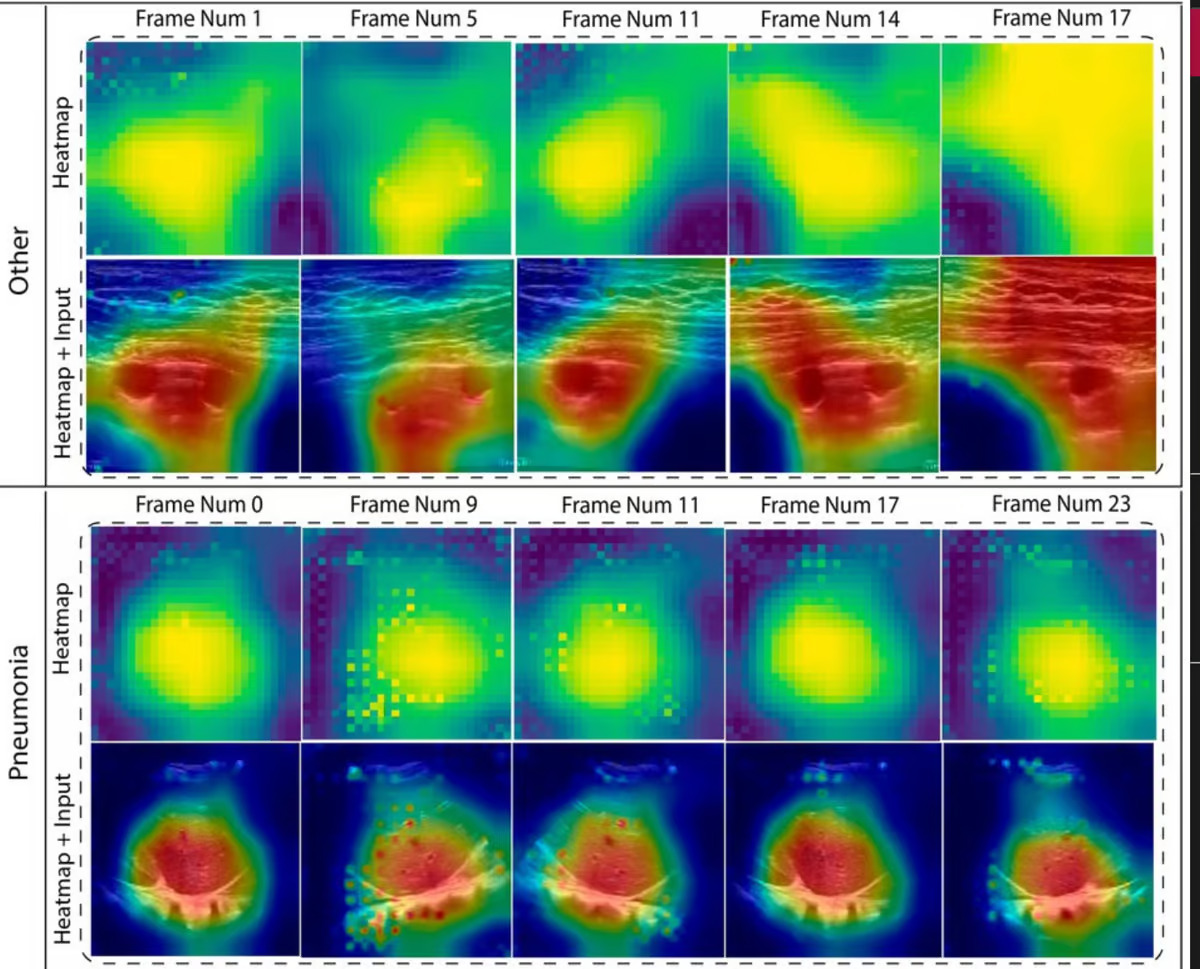
While there’s little doubt that AI diagnostics will soon be commonplace in clinics, skepticism and distrust of this emerging technology remains. While the AI chatbots we can interact with now are not trained to clinically assess medical scans or tests at this stage, specific models are being developed across the board to be reliable tools in healthcare.
For example, just a year ago the Food and Drug Administration (FDA) approved the use of the DermaSensor device, the first smartphone AI-powered device that was shown to be able to detect around 200 different kinds of skin cancers. (Incidentally, I had a basal cell carcinoma identified through a similar smartphone camera device and AI software.) While the tools are not intended to replace medical professionals – my skin-cancer specialist correctly identified the cancerous spot on my back before using the AI camera device – they are set to become the most beneficial and affordable technology we’ve ever seen in medicine.
This new lung disease AI model gives us a clue as to what is yet to come, with it able to correctly identify nuances that differentiate, for example COVID-19 from pneumonia. As the researchers noted, both of those conditions appeared similar to the human eye, but had distinct patterns that enabled the AI model to spot the difference. It would then produce a report as to why it came to its conclusion for each scan.
“The explainability of the proposed model aims to increase the reliability of this approach,” said Shafiabady. “The system shows doctors why it made certain decisions using visuals like heat maps. This interpretation technique will aid a radiologist in localizing the focus area and improve clinical transparency substantially.”
In 2024, huge strides were made by Google in the area of medical diagnostics and AI. Similarly, the technology is being developed for healthcare to assist with everything from surgery to drug discovery. It’s already shown its potential for use in detecting brain tumors and other cancers.
Shafiabady noted that as long as the model is trained on the right data, it has the potential to further its lung-disease diagnostic abilities, picking up signs of tuberculosis, black lung, asthma, cancer, chronic lung disease and pulmonary fibrosis. And the researchers hope to adapt the model to be able to accurately assess more than ultrasounds, such as CT scans and X-rays.
The research was published in the journal Frontiers in Computer Science.
Source: Charles Darwin University View gallery – 3 images
–
Tags