Residual tumors can cause a wide range of post-procedure complications, and impact patients’ quality of life. Milad Fakurian / Unsplash –
Developed by University of Michigan and University of California San Francisco researchers, the technology is called FastGlioma – incorporating the term ‘glioma’ that refers to a brain or spinal cord tumor.
“The technology works faster and more accurately than current standard of care methods for tumor detection and could be generalized to other pediatric and adult brain tumor diagnoses,” said neurosurgeon Todd Hollon, a senior author of the paper detailing FastGlioma’s effectiveness that appeared in Nature. “It could serve as a foundational model for guiding brain tumor surgery.”
With most tumor removal surgeries, it’s difficult to tell healthy brain tissue and tumorous tissue apart – and as a result, a bit of residual tumor could remain in the cavity from where the mass was removed.
That can lead to any of several complications, including seizures, infections, headaches, cognitive deterioration, and motor dysfunction.
Now while these residual tumors can be located using MRI imaging or a fluorescent imaging agent, they’re not always accessible during surgery, or applicable to all types of tumors.
There have also been other attempts to tackle the problem of hard-to-spot residual tumors, like the laser-equipped SpectroPen from 2010, and gold nanoparticles for imaging during surgery back in 2012. However, it isn’t clear if these were successfully commercialized.
FastGlioma could find greater success, given that the AI-powered diagnostic system only requires access to the open source model and compute power.
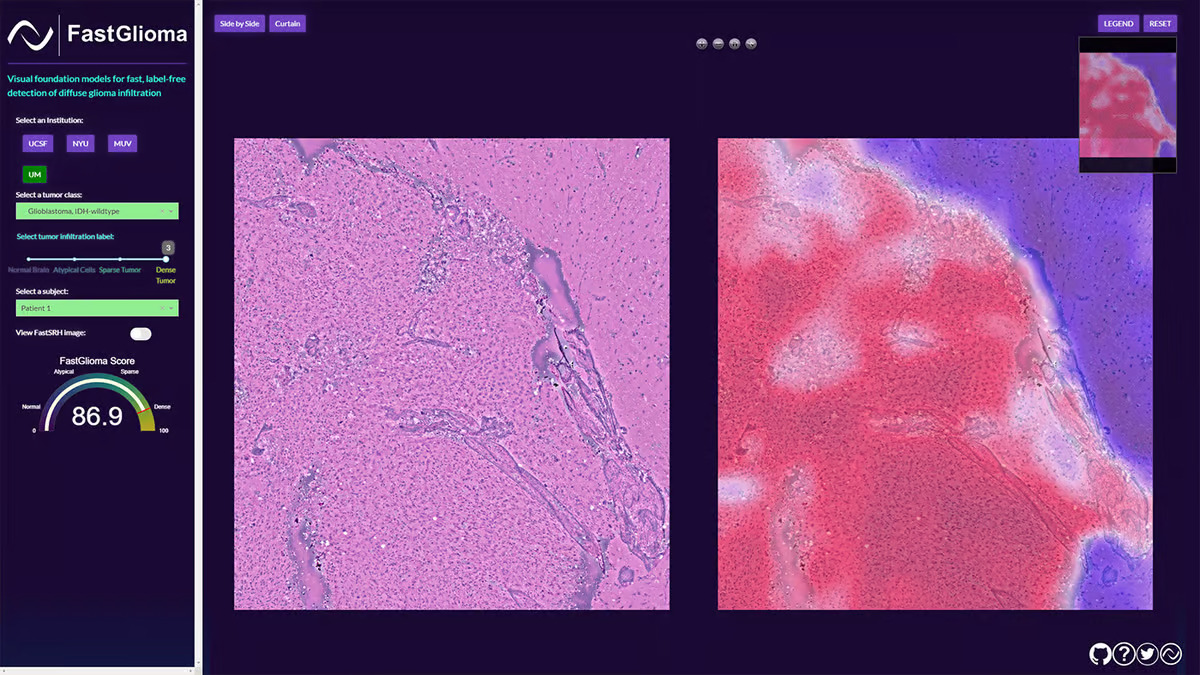
FastGlioma uses foundation models, a type of artificial intelligence system trained on large datasets for a variety of tasks. They can learn patterns to understand language and classify images, with OpenAI’s GPT-4 being a recent example.
In this case, FastGlioma was pre-trained using more than 11,000 surgical specimens and 4 million unique microscopic fields of view. The tumor specimens it looked at are imaged through a high-resolution imaging method called Stimulated Raman Histology.
That enables the system to detect tumor infiltration in just 100 seconds using full resolution images with 92% accuracy. When using lower resolution images, FastGlioma achieved 90% accuracy in just 10 seconds. That enables surgeons to quickly determine if there’s any residual tumor to be removed during a procedure.
This tech represents the biggest advancement in improving the rate of identifying residual tumors in the last two decades. It could help drastically improve patients’ quality of life post-surgery as it gains traction, and reduce the need for costly corrective procedures after the fact.
It’s also a prime example of AI helping to improve patient outcomes in neurosurgery. Last year, we wrote about CHARM, a tool to accurately decode a tumor’s genetic makeup and determine next steps quickly enough to be used during procedures.
FastGlioma could also be expanded to help other types of patients in the near future too. “In future studies, we will focus on applying the FastGlioma workflow to other cancers, including lung, prostate, breast, and head and neck cancers,” said Aditya S. Pandey, who co-authored the paper on the tech.
Source: University of Michigan via Science Daily
–